- AI-based solutions for Continuous Learning
- AI solutions in healthcare: state of the market
- AI: main areas of development
- How the creation of healthcare AI solutions is linked with the work of a human brain
- The problem of catastrophic forgetting
- Training of healthcare AI systems
- Reinforcement Learning technology
- Reinforcement Learning: the model-based and model-free approaches
- Reinforcement Learning algorithm in medicine
- Drawbacks of Reinforcement Learning technology
- Conclusions
AI-based solutions for Continuous Learning
According to The Future Health Index 2021, higher investment in Artificial Intelligence technologies, together with the development of predictive and decision-making tools based on Machine Learning algorithms, is a leading trend in the advancement of the healthcare system. We will tell you about the forecasts for the market of healthcare AI solutions for Continuous Learning and the main areas of development in this field.
AI solutions in healthcare: state of the market
According to research by MarketsandMarkets, the global AI market in healthcare is expected to grow from $4.9 billion in 2020 to $45.2 billion in 2026, with a compound annual growth rate (CAGR) of 44.9% for the forecast period.
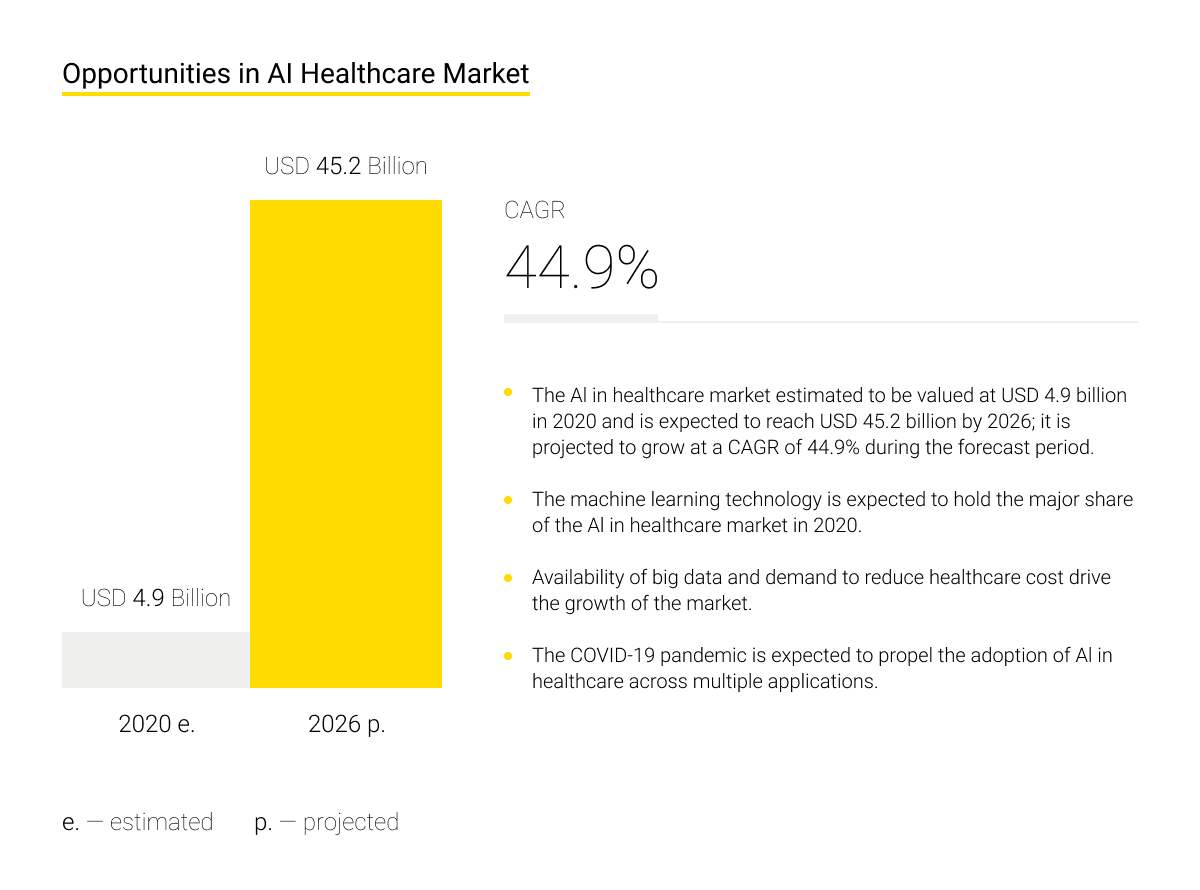
The primary focus is on the development of software for healthcare AI: platforms, applications, software solutions for devices, and cloud technologies. Among the leading areas of AI implementation in healthcare are Computer Vision and Natural Language Processing for diagnostics.
Then comes patient data analysis, risk management systems, Inpatient Care & Hospital Management systems, drug research, and the development of virtual physician assistants that provide patient care without the need for human specialists.
Among the leading regions for the development of AI for healthcare are North America, Europe, and the countries of the ASEAN region with a predominance of American high-tech companies in the market.
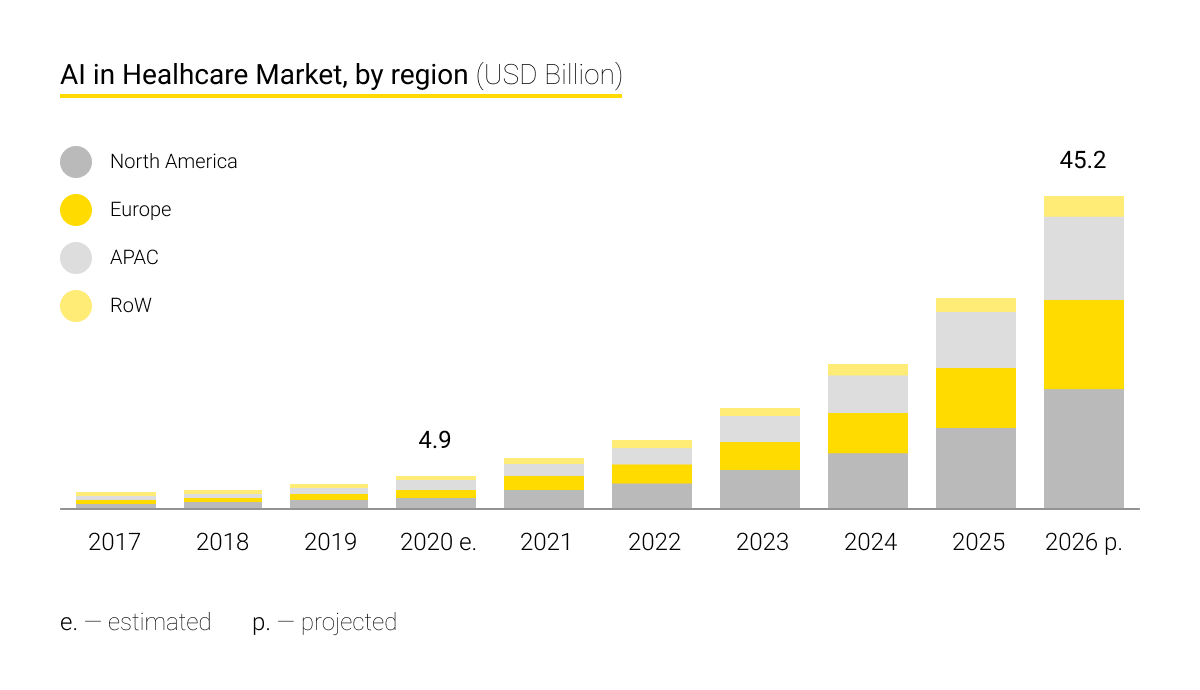
IBM heads the list of leading players in the market. The corporation has 12 research laboratories at its disposal and over 1,400 patents in the field. The research also names such companies as NVIDIA Corporation (US), Intel Corporation (US), Google Inc. (US), Microsoft (US), Amazon Web Services (US), Siemens Healthineers (Germany), Koninklijke Philips N.V. (Netherlands), and others.
AI: main areas of development
Aiming for the widespread introduction of AI tools into businesses and key sectors of economic and social advancement is beyond doubt - this is supported by the results of global surveys by business leaders: Accenture, Gartner, and Deloitte. Machine Learning (ML) technology, its advantages, and advancement problems attract the most interest.
ML is the general name for a number of technologies, the purpose of which is to create models that can autonomously cope with resolving complex tasks. Applying Reinforcement Learning technologies has a tangible impact on the healthcare sector, so the rest of this article will be devoted to them.
There are two main areas in the development of AI.
The first one is aimed at creating locked AI, trained to perform tasks within an artificially limited environment with maximum typification and automation of objects and procedures. This approach has proven itself in solving Computer Vision tasks, compiling registries, etc.
The second one focuses on forming AI as a (semi)autonomous agent capable of acting and developing in a dynamic complex environment. This presupposes the readiness of the system to learn in the course of interaction. Something like this happens with human intelligence, the performance and capabilities of which increase as new experience is accumulated and new skills and intellectual procedures are learned.
How the creation of healthcare AI solutions is linked with the work of a human brain
Continuous Learning technology strives to ensure that AI doesn’t remain restricted by its original experience but constantly improves while it is functioning. This is about shaping AI as an autonomous agent working with real-time data. We may think about analogies concerning the organization of the corresponding processes in human intelligence: is there a possibility to directly transfer its methods of organization and functions?
In the case of human intelligence, neurocognitive mechanisms influence the formation, advancement, and specialization of skills, as well as long-term memory capabilities. The use of similar approaches for creating and training artificial neural networks is associated with the accumulation of constantly updated information. This leads to interference, also known as catastrophic forgetting - as new input data is received, the system begins to forget the old one, and its skills become unstable and dependent on current experience.
To ensure that intelligence, whether human or artificial, is capable of storing and correctly processing large volumes of information while maintaining the stability of the main functions, it is necessary to choose harmonious values for the parameters within the framework of solving the stability-plasticity dilemma. In childhood, human intelligence is the most flexible, but over time, the ability to learn quickly decreases in favor of the stability of basic thought processes and memory management. At the same time, some degree of plasticity is preserved - experience is accumulated and affects behavior and thinking.
There are two types of plasticity working in a human brain:
- Hebbian plasticity, referring to the strengthening of connections between neurons with the intensification of their interactions, and
- compensation homeostatic plasticity with the stabilization of neuroactivity, which is provided by the dynamics of feedback mechanisms and changes in the activity of synapses; as part of this, stable connections that are in demand in further experience are fixed, while others are weakened.
Together, these types of plasticity ensure the stabilization of neural circuits to form optimal connectivity and functionality patterns based on previous experience.
The second component responsible for the neurosynaptic adaptation mechanism, along with the above-mentioned plasticity, is the joint work of the brain’s different areas: the hippocampus and the neocortex. This phenomenon is described by the Complementary Learning Systems (CLS) theory. What happens here is an alternation of mechanisms for fast learning based on short-term memory and the generalization and structuring of knowledge using long-term memory.
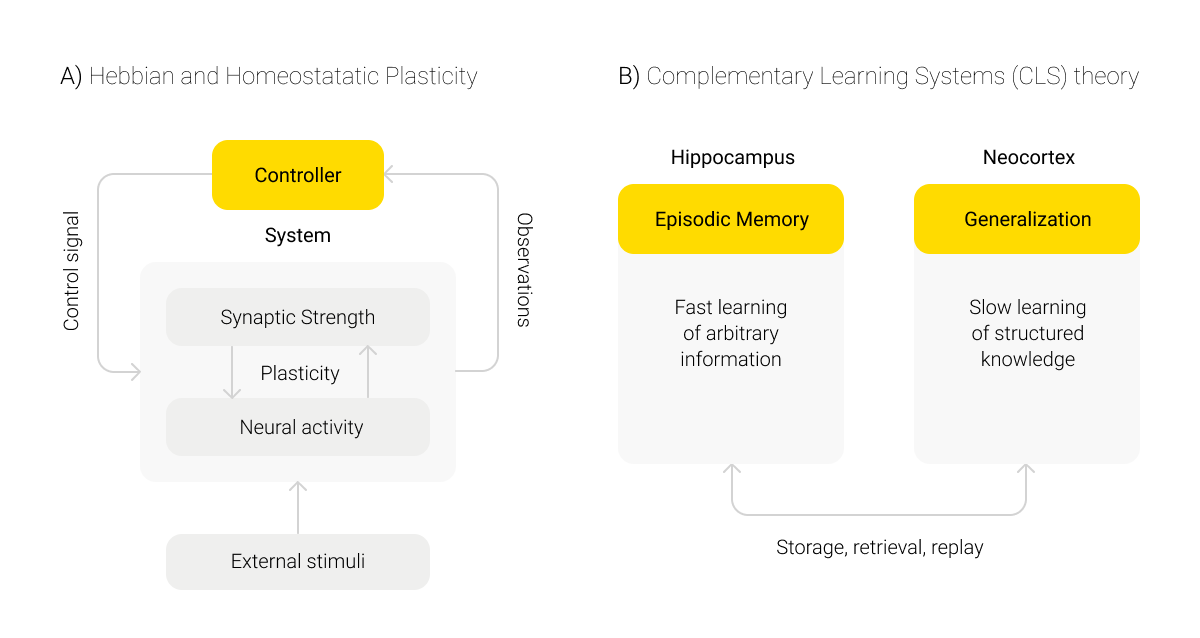
Certain patterns of human memory functioning are applied when developing mechanisms for ML and neural network training. However, the differences between artificial and organic intelligence go beyond process architecture and engage the interaction of these systems with external stimuli.
For example, in the case of human intelligence, the problem of interference is not so acute because the experience data is interconnected and closely intersected. From an early age, our brains learn to process more and more complex information for the gradual improvement of the basic functions of perception, cognition, and behavior. Moreover, human intelligence uses the data of its space-temporal experience much more strongly when building blocks of memory, integrates the results of acquired and passively acquired experience, and generates an extensive field of associative links.
Most AI systems, in turn, train on the basis of standard data sets repeating many times in random order. Fast recognition of information and prediction of new combinations within the used algorithm are effectively applicable in image recognition programs but not in Continuous Learning.
The problem of catastrophic forgetting
Continuous Learning largely uses the task-incremental learning (Task-IL) scenario, as well as class-incremental learning (Class-IL). This raises a question about the ability of the system to solve the data security problem and transfer experience gained during solving one class of problems to problems of another class. One of the methods for working out this scenario without having to allocate significant resources for data storage is the method of Generative replay. However, the problem of catastrophic forgetting remains a considerable disadvantage of GR.
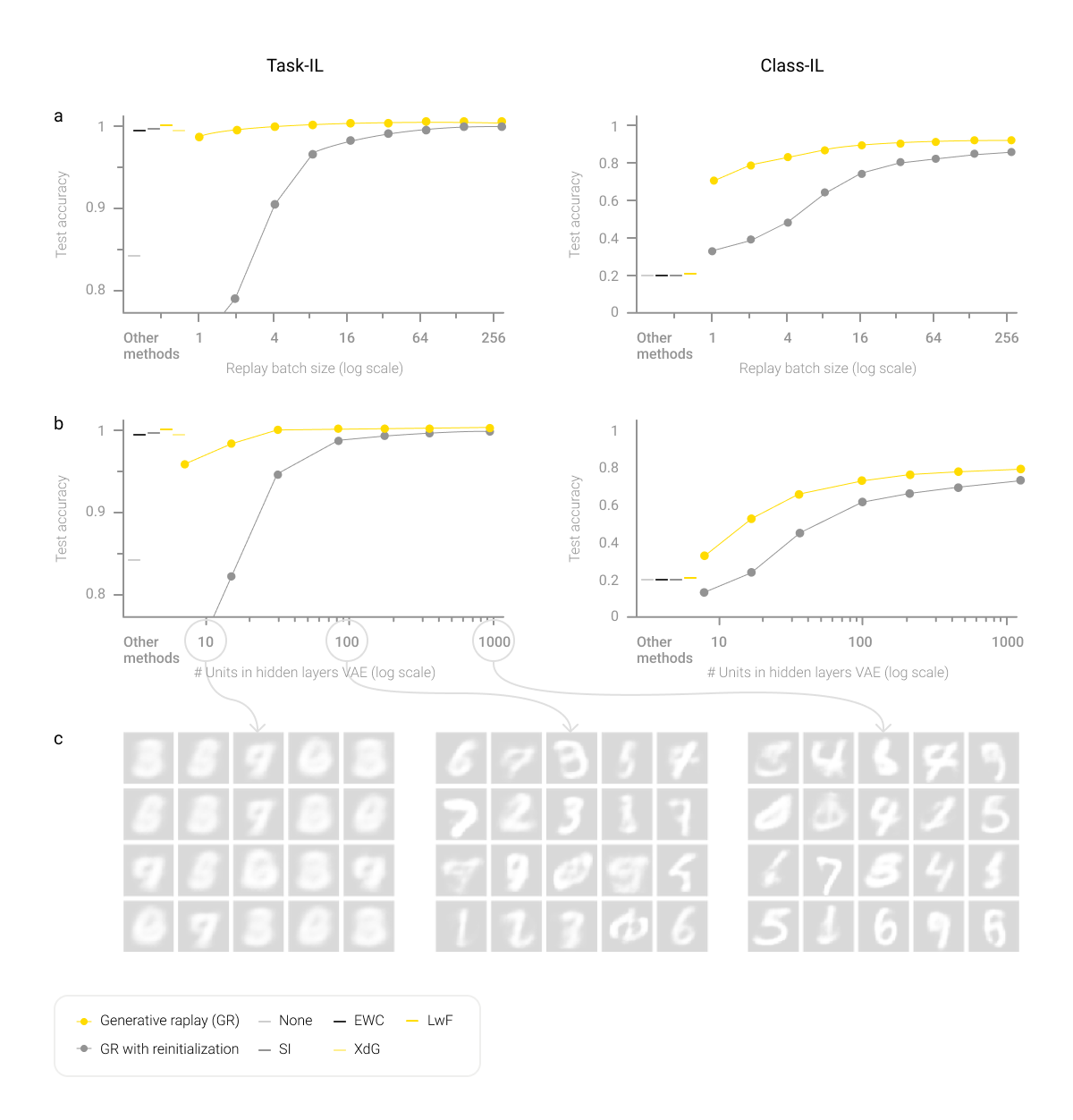
The problem of interference, first described by McCloskey and Cohen in their 1989 study, is central to ML algorithms. It queries the interaction of parameters obtained throughout the learning process with data obtained from the execution of previous tasks, as well as with the data generated to perform new tasks.
Classical methods of solving the problem of catastrophic forgetting are Feature Extraction, Fine-tuning (original and duplicated), and Joint Training. The difference between them is determined by different approaches to optimizing and organizing the interaction between the parameters of neural networks.
The common essence of these methods is to find the balance between the performance of resolving old and new tasks, the efficiency of training and subsequent testing, and the required amount of resources for storing data. As you can see from the table below, with an enhancement in task resolving performance, the need for data storage resources increases, and the efficiency of training or testing algorithms decreases.
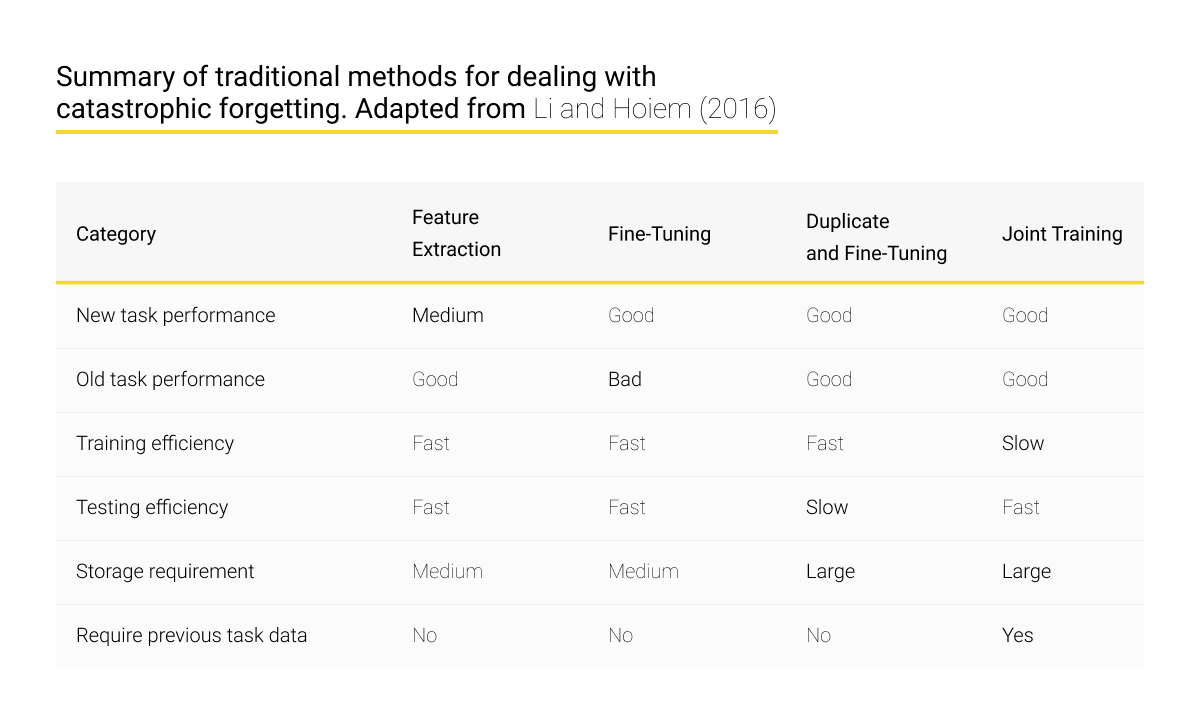
Training of healthcare AI systems
The above approaches are mainly organized as part of supervised learning, during which a developer’s participation and control over the state of AI systems and their performance are maximized. However, for greater autonomy and improving the efficiency of AI interaction with the environment, unsupervised learning algorithms are being introduced. They are usually used as part of Continuous Learning technologies.
Quite often, AI systems are trained on the basis of such widespread data sets as MNIST, CUB-200 (Caltech-UCSD Birds 200), CIFAR-10 and CIFAR-100, SVHN (Google Street View House Numbers), CORe50, and others. Each of them is a database with digits, symbols, or images of objects belonging to a certain class, the recognition and classification of which the current algorithms for neural network training are being configured for.
Among options for implementing unsupervised learning technology, we should highlight such methods as Learning without Forgetting, Elastic Weight Consolidation, Progressive Neural Networks, the autoencoder model, and Reinforcement Learning.
Learning without Forgetting (LwF) is a Continuous Learning model that focuses on memorizing previous parameters to improve performance on old tasks. However, it requires significant data storage resources and may not apply updated algorithms for old tasks, even if they are more efficient.
Elastic Weight Consolidation (EWC) is a training method where the functioning of synaptic links is imitated in such a way that the plasticity of the system decreases when working with already learned tasks and remains increased in the case of performing new tasks. Therefore, the weight of the gained experience works for stabilizing the system's links and for memorizing them by smart algorithms - the only parameters saved are those that are beneficial for AI when resolving learned types of problems.
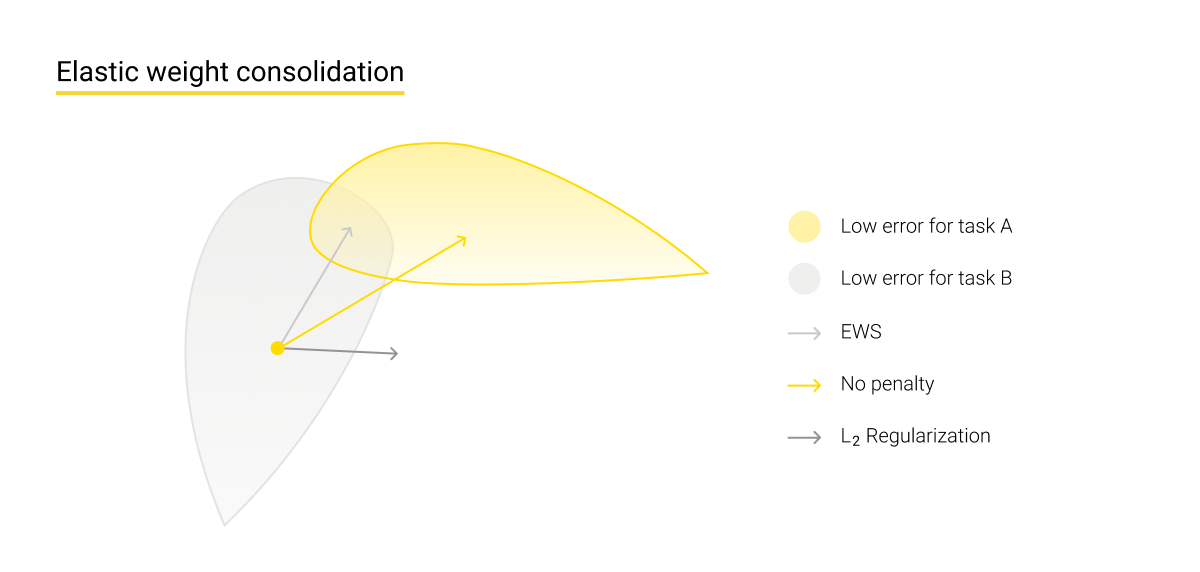
The Progressive Neural Networks model, which is widely used as part of both supervised and unsupervised learning, presupposes using a set of pre-trained models as knowledge of the system, connected by a network of hidden links. This allows AI to apply various combinations of these models to resolve new tasks, which, however, is limited by the existing “knowledge” set. This method is also similar to the Network of Experts model, where each algorithm is trained to cope with a certain type of problem at the expert level but transfers control to other algorithms when a task nonrelevant to it appears.
The autoencoder model presupposes a neural network structure where the input data is also restored at the network output, i.e. an encoder and a decoder are united into a single system. Thus, the algorithm remembers the previous parameters but only applies those that have proven their effectiveness at this stage. This model can also be used in conjunction with LwF in order to preserve only those system properties that are necessary to perform previous tasks and modify other properties to resolve new tasks.
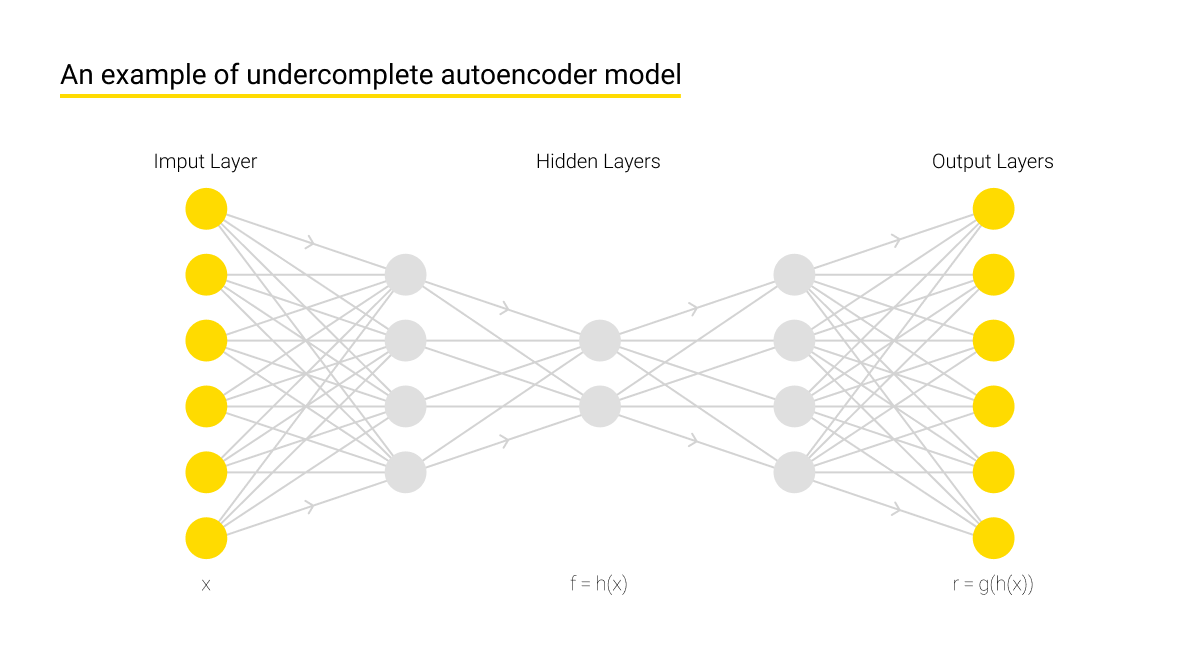
Reinforcement Learning technology
Finally, special attention should be paid to such a method of Deep and Continuous Learning as Reinforcement Learning, which is widely used in healthcare.
This approach uses a Continuous Learning agent called Unicorn. An agent of a neural network is an actively running process that performs specific sequences of tasks. This agent is able to simultaneously learn a variety of tasks, including new ones, and use the accumulated knowledge to effectively resolve suitable tasks, particularly but not exclusively by finding deep and unobvious dependencies.
One of the advantages of this approach is learning multiple tasks in the off-policy mode. This means that performing a task in the mode of a separate policy (action strategy for an agent to resolve a specific task) can change the strategy for performing other tasks as a result of the gained experience, which makes the system extremely dynamic and highly adaptive.
The algorithm itself is built around the actions of an agent that, depending on the efficiency of the task, can receive a reward as part of implementing the scenario regarding interaction with the environment. Also, along with the short-term reward, the system can accumulate a long-term value parameter. This parameter summarizes the effect obtained from a set of actions and influences the change in the final action policy (π) of the agent.
There are three basic models for executing a Continuous Learning algorithm:
- Value-based, where the key emphasis is on maximizing the value function within the current state and the particular strategy used;
- Policy-based, existing in deterministic and stochastic versions, presupposes an emphasis on choosing an action policy (either rigid or determined by the sum of circumstances) that would bring the maximum result from the performance of any action in the future;
- Model-based, where the focus is on the agent’s interaction with the environment and its thorough study through virtual training models.
Reinforcement Learning includes the positive and negative ways of reinforcing actions. In the former case, result-oriented actions are rewarded, which can lead to an increase in the system performance but often leads to unreasonable optimization of actions, when AI ignores other opportunities besides the reinforced ones. In the latter case, the minimum survival strategy for an especially rough environment is ensured, when a performance limit, with which the task will be considered minimally achieved, is set.
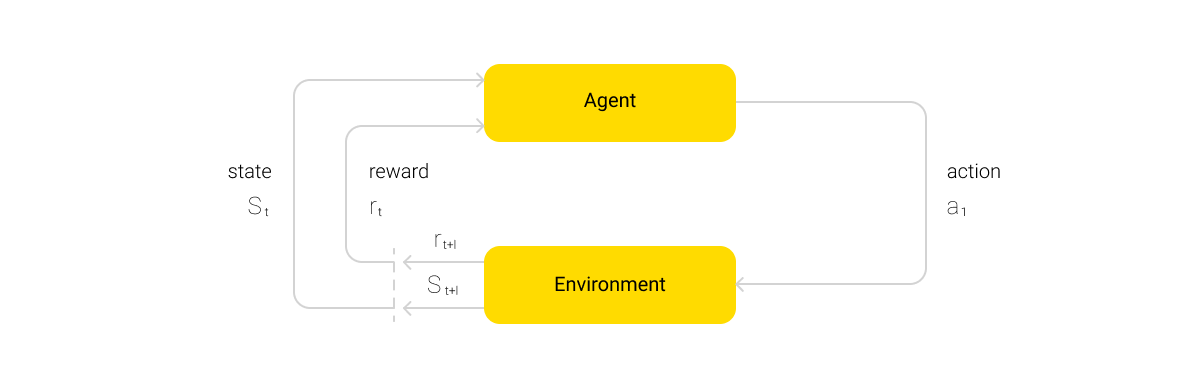
Reinforcement Learning: the model-based and model-free approaches
Reinforcement Learning can be conducted in either model-based or model-free format. In the latter case, the algorithm works directly with the value of action parameters to optimize performance without having an environment model. This mostly means tactical benefits despite strategic advantages. This is similar to an approximate algorithm for the actions of our ancestors who had no idea about the world structure but worked on solving specific problems to survive.
Statistically, this approach is less effective. It requires the system to constantly evaluate and correlate the value of actions based on information about the world, often inaccurate and distorted, without the possibility to rely on a global understanding of the environment for direct action. The most common method of model-free Reinforcement Learning is the Q-learning method.
The basic method of the system’s interaction with the environment is the probability model of Markov decision process (MDP). In this case, when performing new problems, accurate data is not available, and the reward system is not obvious. The model is widely applicable in various Deep Learning processes. The Q-learning algorithm helps to find the best strategy for maximizing the expected value of the total reward at all successive steps, starting from the current state, for each end MDP. This allows the neural network agent to operate effectively in an unfamiliar world, focusing on a limited set of environmental parameters.
The model-based approach, on the contrary, is based on the assumption that the system has a general knowledge of the world and the ability to strategically plan and rank its actions on the basis of existing knowledge. Although the model-based approach is better at helping algorithms interact with the environment and perform tasks more accurately, it’s usually limited to certain types of tasks built into the model.
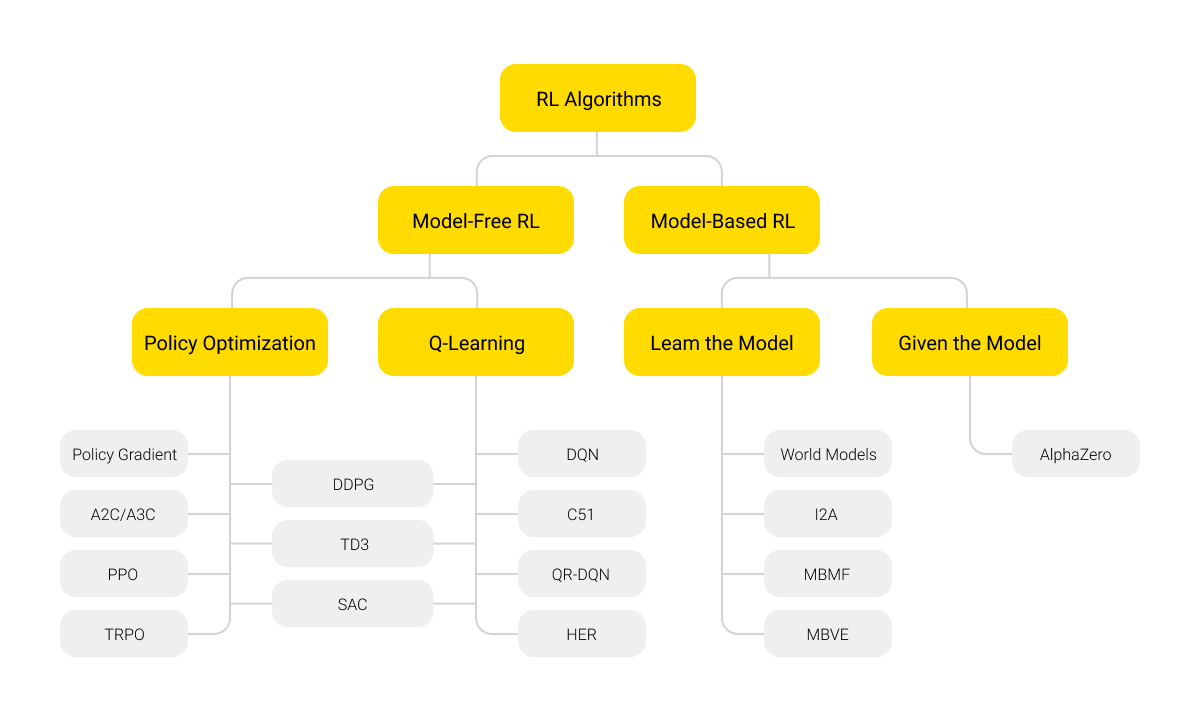
Reinforcement Learning algorithm in medicine
Reinforcement Learning algorithms are applied in various fields - for example, in computer games, automatic and robotic systems, business planning, and the creation of self-learning chatbots, including medical ones. Their advantage is obvious when used in the field of medicine and healthcare.
If you look at a detailed survey concerning the use of Reinforcement Learning in creating software for medical devices, you will see how wide the current range of applying AI in healthcare is. The advantage of this approach over other ML technologies is determined by the capability of Reinforcement Learning to find the best possible strategy based on data from previous experience without necessarily relying on a complex mathematical model of a biological system.
As such, Reinforcement Learning is used in clinical decision support systems - for example, determining dynamic treatment regimens, automated medical diagnosis, as well as optimizing management and control processes, resource management, health management, and drug research.
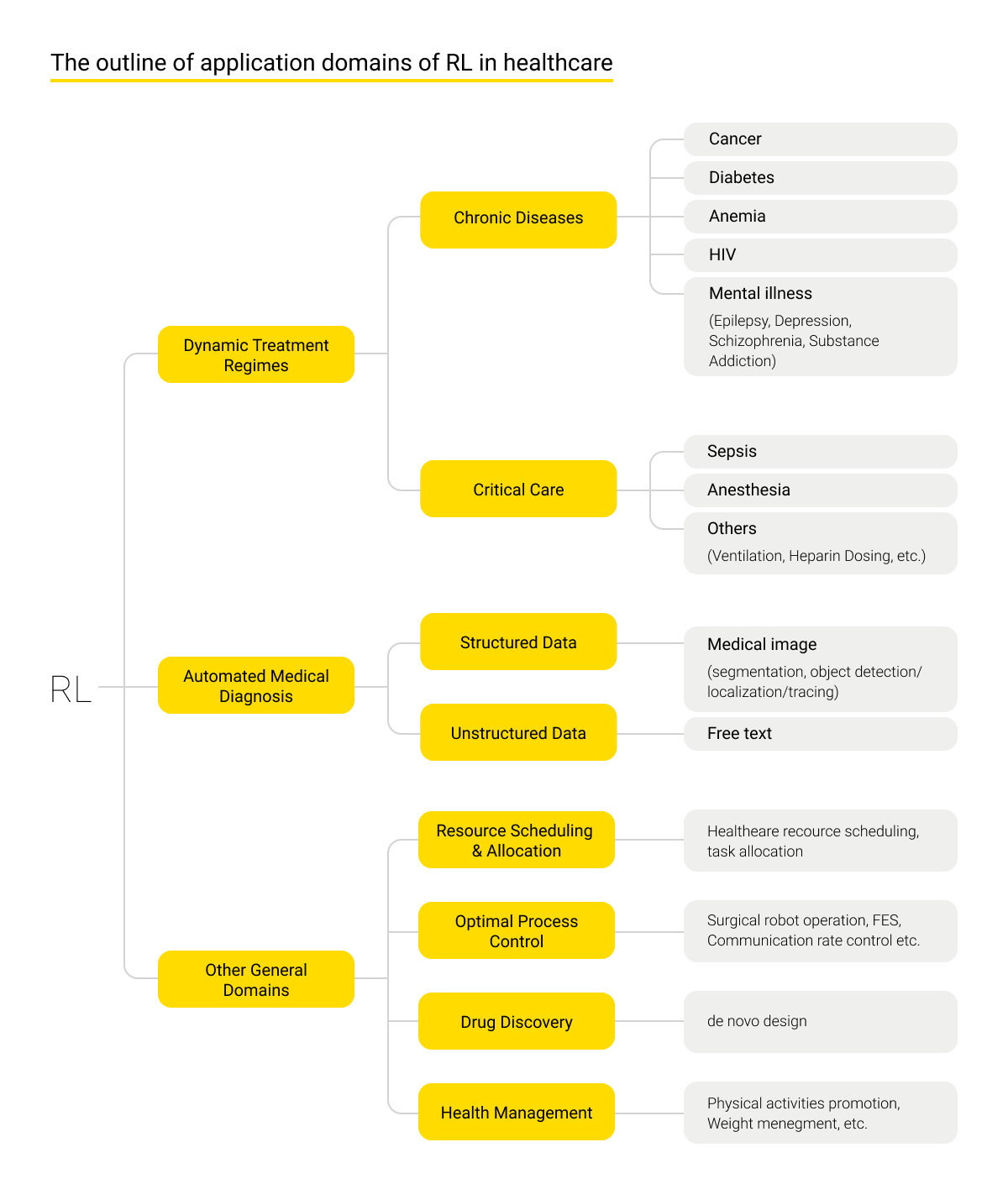
Dynamic treatment regimens (DTRs), also called adaptive interventions or adaptive treatment strategies, refer to a paradigm for automating the development of new effective treatment regimens for individual patients undergoing long-term care. Notable results have been obtained when treating a range of chronic diseases like cancer, diabetes, anemia, AIDS, and mental illnesses, as well as when performing clinical treatment for sepsis, carrying out anesthesia procedures, etc. For example, such model-free algorithms as Q-learning and Temporal Difference are used in chemotherapy for treating cancer patients.
Reinforcement Learning algorithms are applied to optimize chronic disease treatment regimens. Such methods include radiotherapy and effective means of continuous blood glucose monitoring, stimulation of the hemoglobin content growth with the help of erythropoiesis-stimulating agents (ESAs), and Highly Active Antiretroviral Therapy (HAART) improvement. The latter two are used to suppress drug-resistant types of HIV using improved Structured Treatment Interruption (STI) strategies.
When emergency care is being organized, Reinforcement Learning algorithms help to establish smooth work with large amounts of data supplied by the emergency department. The algorithms find treatment policies that will stabilize essential vital functions in the most effective way. This affects the decision-making mechanisms for dealing with sepsis, decisions on sedation and pain relief, and other cases.
These algorithms are also applied for image and speech recognition as part of automatic diagnostics procedures, which is especially important for reading examination data and their automatic identification. A number of improvements can also be achieved by applying these algorithms for planning resource use and clinical management, enhancing the performance of surgical robots, accelerating the creation and testing of new drugs, developing recommendations for prophylactics and treatment of patients at the recreational therapy stage, etc.
Drawbacks of Reinforcement Learning technology
Like Continuous Learning technology in general, Reinforcement Learning technology is not free from problems and shortcomings, despite the aforementioned strides, and both developers and end-users should bear them in mind.
In the case of the model-based approach, preparing a model that simulates the real environment is of special significance. This is easy to provide, for example, when simulating a chess game, but problematic for a complex dynamically organized environment, for instance, for an automated car driving program, as there is a danger of ignoring other factors. Therefore, when transferring the trained model to the real world, various inconsistencies are possible - for example, the real clinical picture of a patient's condition is more complicated than the simulated situation.
Scaling and configuring an artificial neural network that controls an agent can also be challenging. This network primarily focuses on a system of rewards and penalties and can selectively remove currently underutilized parameters, which brings us back to the problem of catastrophic forgetting. One of the major challenges is how to seamlessly detect, integrate, organize, and resolve different challenges or tasks with different levels of detail in one or more networks with minimal interference.
The problem of achieving a local optimum and incorrect transfer arises when the managing agent opts for one strategy that gives the maximum result in most cases, ignoring the most effective strategies for resolving a specific task. An example is when the agent uses a proven general scheme for reducing pressure in a patient while there is a need for a different approach determined by important concomitant circumstances: individual intolerance, drug incompatibility, and other threats.
The pursuit of efficiency in the form of maximizing rewarded results can lead to deviations from the optimal strategy in order to increase the “profit” for the system. This can result in prolonging the treatment procedure for the sake of 100% problem solving instead of moving to the next stage. For example, taking into account the natural process of body regeneration after the critical threat is combatted, treatment procedures presuppose the possibility of switching to the treatment of other conditions where the threat is higher, not a complete neutralization of that one. The AI algorithm can ignore this, seeking to work on the current stage as much as possible.
Selecting representative data and strategies can become a problem when there are no clear criteria for separating distorted and inaccurate data from adequate ones. The influence of the context can exceed the current conditions of the task execution, which affects the quality of the AI’s functioning.
Conclusions
Despite the challenges and problems in the implementation of Reinforcement Learning and Continuous Learning in general, it’s a promising ML technology for healthcare advancement. A certain degree of reliability and compliance with security standards when using ML technologies is guaranteed by certifications from the US Food and Drug Administration (FDA), European Medicines Agency (EMA), Conformité Européenne (CE) marking, the National Medical Products Administration (NMPA), and other organizations.
The advancement of Continuous Learning and, in particular, Reinforcement Learning systems in the healthcare sector will grow rapidly. This is indicated by the dynamics of the AI market in healthcare, the willingness of business leaders to introduce healthcare software solutions, and a large number of leading development companies. All this will lead to an uptick in the availability and quality of medical care.
Further advancement of the technology, overcoming the catastrophic forgetting problem, and solving challenges and technical problems will accelerate the development of AI-based products in medicine and healthcare, both at the level of clinics and hospitals and for patients and ancillary medical institutions. All this will create new opportunities for healthcare software development companies.